INSIGHTS
Meeting the Infrastructure Demands of Generative AI
Altman Solon, the largest global strategy consulting firm dedicated to the TMT sectors, examines how generative AI impacts network infrastructure. The final article in the “Putting Generative AI to Work” series examines the impact of emerging enterprise generative AI solutions on compute, storage, and networking infrastructure.
The best spokesperson for generative AI might be ChatGPT. The chatty, engaging app, developed by OpenAI, dazzled millions worldwide with life-like answers to any number of questions. Going under the hood, OpenAI’s impressive large language model (LLM) runs on a vast supercomputing infrastructure. In the case of ChatGPT, OpenAI relied on their partner Microsoft Azure’s cloud computing platform to train this model. Microsoft spent years linking thousands of Nvidia graphics processing units (GPUs) together while managing cooling and power constraints.
Altman Solon believes growth in enterprise-level generative AI tools will lead to incremental demand for compute resources, positively benefiting core, centralized data centers, where training occurs, and local data centers, where inference occurs. Network providers should see a moderate increase in demand for data transit networks and a boost for private networking solutions.
We used a four-step methodology to understand infrastructure impact, accounting for the average compute time requirement per generative AI task, the volume of overall generative AI tasks, the incremental compute requirements needed, and the quantifiable impact on the infrastructure value chain. To meet this demand, service providers will need to start planning for adequate compute resources and network capacity.
Quantifying enterprise generative AI use cases
Using data from our survey of 292 senior business leaders, Altman Solon calculated the hourly volume of generative AI tasks across four business functions – software development, marketing, customer service, and product development/design. Considering the potential adoption rate per use case, average users per case, and average generative AI tasks per user hour, we estimate between 80-130 million generative AI tasks per hour within the next year across these key enterprise functions in the US alone.
Generative AI Tasks per Hour Across Enterprise Functions
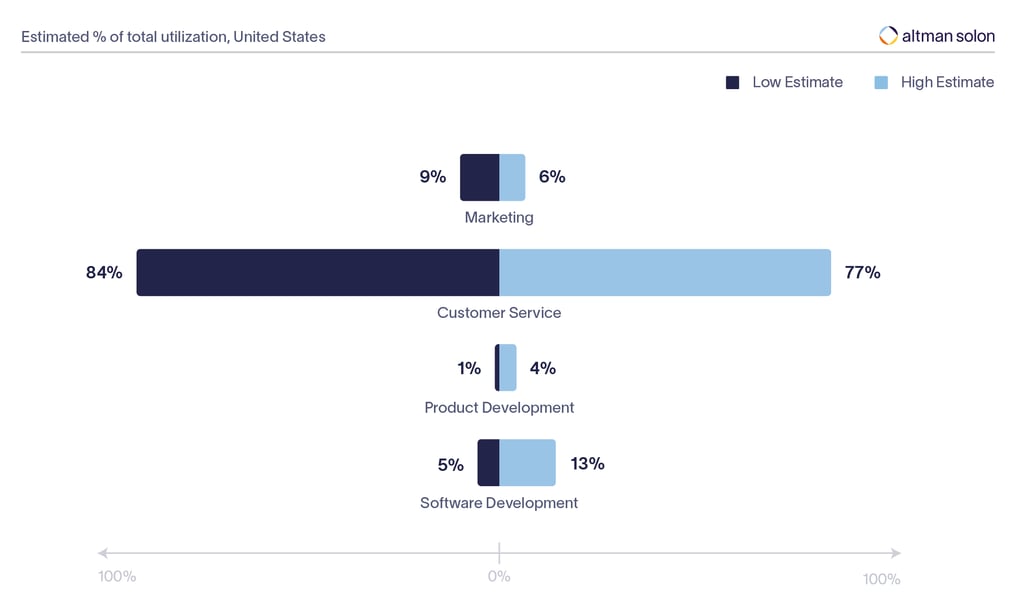
Although our research shows that customer service has been slower to adopt generative AI solutions than software development and marketing, customer service tasks would be responsible for the bulk of generative AI usage for enterprises. This is primarily due to the sheer volume of user interactions with generative AI chatbots. Of course, computing doesn’t only happen when an LLM is queried. Building and using generative AI requires considerable infrastructure.
Developing and using generative AI solutions: training and inference’s infrastructure impact
When building and using generative AI tools, compute resources are required during two distinct phases: training the model and then using the model to respond to queries (also known as ”inference”). Training an LLM entails feeding a neural network vast amounts of collected data. In the case of ChatGPT, large amounts of text data were inputted to the LLM to recognize patterns and relationships between words and language. As the model processed new text data, its performance improved, and the parameters or values assigned to neural connections changed. The GPT-3 model contains over 175 billion parameters; GPT-4 is speculated to have 1 trillion parameters.
Training a Large Language Model: From research to human feedback
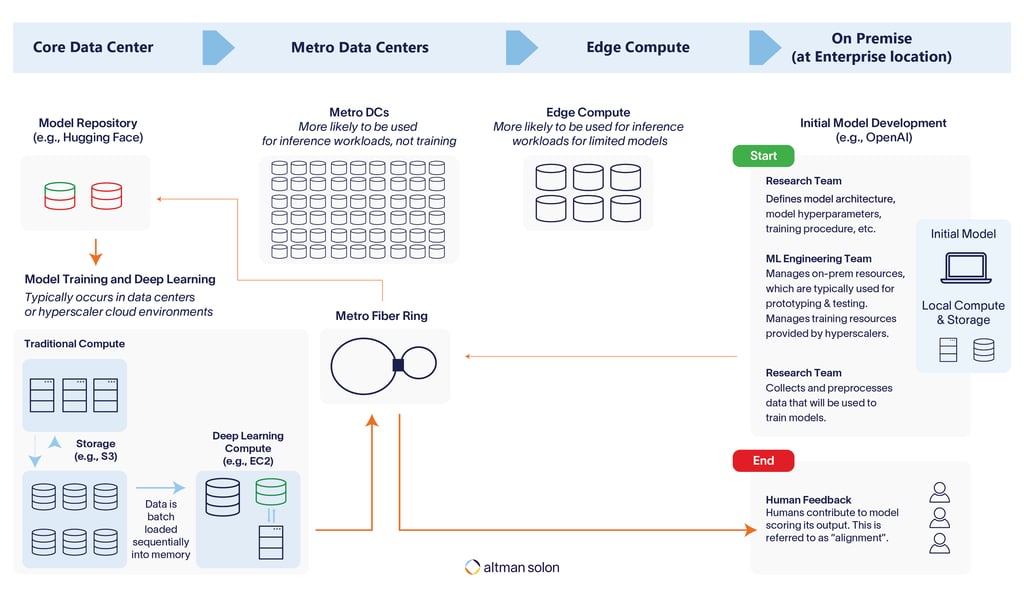
Even though iterating massive amounts of data to train a model is compute-heavy, training occurs only during model development and stops once the model is finalized. Over an LLM’s lifespan, training will take up 10-20% of infrastructure resources. By contrast, inference is where most workloads take place. When a user enters a query, the information is fed through the generative AI application’s cloud environment. 80-90% of compute workloads occur during inference, which only increases with use. To use the example of ChatGPT, training the model supposedly costs tens of millions of dollars, but running the model is reported to exceed the training costs weekly.
Housing and training generative AI solutions: Compute impact to be felt most by public clouds and core, centralized data centers
Generative AI models tend to be trained and stored in centralized, core data centers because they have the GPUs necessary to process large amounts of data that cycles through LLMs. Models are also housed and trained in the public cloud to eliminate ingress and egress costs since training data already resides there. As adoption grows, we expect inference to be conducted more locally to alleviate congestion in the core, centralized data centers where models are trained. However, this has some limits: generative models are too large to house in conventional edge locations, which tend to have higher real estate and power costs and cannot be easily expanded to accommodate resource-hungry AI workloads.
“We built a system architecture [for the supercomputer] that could operate and be reliable at a very large scale. That’s what resulted in ChatGPT being possible,” she said. “That’s one model that came out of it. There’s going to be many, many others.”
— Nidhi Chappell, Microsoft General Manager of Azure AI infrastructure —
We believe the uptick in generative AI tools will thus have the most significant impact on the public cloud. Public cloud providers should focus on regional and elastic capacity planning to support demand. In the near future, as models mature and as critical compute resources become cheaper, private cloud environments might begin housing and training LLMs. This could be particularly useful for industries in regulated spaces like pharmaceuticals, financial services, and healthcare, which tend to prefer developing and deploying AI tools on private infrastructure.
Querying generative AI tools: Network impact to be felt most by backbone fiber and metro edge networks
Network usage of generative AI tools is affected most by query volume and complexity. For example, asking ChatGPT to answer a fundamental question versus asking it to draft a 900-word essay impacts network usage differently, just as requesting a single image on Dall-E versus a series of high-definition photos or videos does. Longer, more complex outputs require higher volumes of data, impacting increasing demand on backbone and metro edge networks. This is especially true for the fiber that connects to major cloud platforms or between cloud environments. We predict that metro edge networks will experience an uptick in usage as generative AI matures and gets mainstreamed by consumers and businesses. The number of queries could create traffic and compute or storage saturation in specific cloud choke points, necessitating a more distributed approach to housing AI models (e.g., replicating in regional cloud environments).
Implications across the infrastructure value chain
Although we are still in the early days of generative AI adoption, we believe that to meet increased compute, networking, and storage demand, infrastructure providers should adapt in the following ways:
- Core, centralized data centers: Model developers rely on cloud providers for their compute needs for training. In the immediate future, data centers should focus on capacity planning to ensure adequate compute, power, and cooling resources for training and inference workloads. In the long run, if generative AI models proliferate and there is an increase in image and video-generating LLMs, there is a real risk of network and compute congestion in core, centralized data centers. Cloud providers can mitigate this risk by providing compute capacity in metro DCs / regional availability zones that can handle heavy generative AI workloads.
Furthermore, demand for core, centralized data centers and cloud services from generative AI is channeled through model providers like Cohere and OpenAI. As such, core, centralized data centers should explore exclusive partnerships, such as the one between Azure and OpenAI, to ensure lock-in. At the same time, to avoid consolidation around a single provider, core, centralized data centers should also pursue open-source partnerships. Integrating LLM technology into PaaS and SaaS business can be a savvy way to facilitate adoption and increase revenue. - Backbone and Metro Fiber: There is an increase in demand primarily driven by training data, inference prompts, and model outputs. There is also a change in traffic topology: model-generated content originates in core, centralized data centers and is produced in real-time. As such, it cannot be pre-stored in locations close to end users. Backbone and metro fiber providers should grow network capacity and egress bandwidth to meet increased demand. In addition, enterprise users are concerned with data privacy and residency, especially when that data is used for training. These concerns will likely lead to increased demand for private WAN solutions, especially WANs incorporating cloud connectivity. Lastly, AI models are increasingly housed with a different cloud provider or in a different compute environment from other enterprise applications and workloads. This leads to latency concerns, which backbone fiber providers can address through interconnects and peering, especially with core, centralized data centers and model providers.
- Metro Data Centers and Edge Compute: While we expect inference to be pushed to metro data center locations, we don’t expect the same models to be pushed to smaller “edge” compute locations. Currently, there isn’t much value (or feasibility) to push inference workloads to the edge because compute latency is still far higher than networking latency, and generative AI hardware is hard to come by in core, centralized data centers, let alone at the edge. However, in the future, smaller generative AI models could be optimized for readily available CPUs in edge data centers. In the meantime, edge compute environments could also target complementary workloads like prompt pre-processing, image or video pre-processing, and model output augmentation.
- Access: Access networks will see relatively smaller increases in demand, as early generative AI traffic more closely resembles search traffic over access networks. Over time, the complexity and volume of generative AI traffic over access networks will increase (e.g., generative video) but will only moderately impact Access bandwidth demand.
- On-Premise: On-premise hardware does not meet the requirements of generative AI models, especially in terms of on-chip memory. While models are unlikely to run on-premise in the foreseeable future, it is an active area of research due to data privacy concerns and the appeal of running LLMs locally on mobile devices.
Using LLMs to automate essential business functions will not only impact employees and management. Still, it will also affect the building blocks of generative AI itself, from core, centralized data centers to original equipment manufacturers, chip manufacturers, and designers. While the future has yet to be written, infrastructure providers should consider how to service a technology requiring considerable computing, storage, and network resources.
About the analysis
In March 2023, Altman Solon analyzed emerging enterprise use cases in generative AI. Leaning on a panel of industry experts and a survey of 292 senior executives, Altman Solon analyzed preferences for developing and deploying enterprise-grade generative AI tools. The survey also examined the key deciding factors in developing and deploying generative AI tools. All organizations surveyed were from the United States of varying business sizes. Explore additional insights from our Putting Generative AI to Work series, including emerging generative AI use cases for enterprises and preferences for developing and deploying enterprise generative AI tools.
What could the adoption of generative AI mean for your business?
Fill out the form below to gain strategic insights into the implications of generative AI use.
Thank you to Elisabeth Sum, Oussama Fadil, and Joey Zhuang for their contributions to this report.